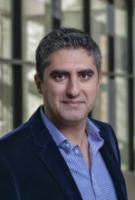
Alireza Doostan is an H. Joseph Smead Faculty Fellow and Associate Professor of Aerospace Engineering Sciences Department at the University of Colorado Boulder. He is also the director of the Center for Aerospace Structures (CAS) and an affiliated faculty of the Applied Mathematics Department. Prior to his appointment at CU Boulder in 2010, he was an Engineering Research Associate in the Center for Turbulence Research at Stanford University. Alireza received his PhD in Structural Engineering and M.A. in Applied Mathematics and Statistics from the Johns Hopkins University both in 2007. He is a recipient of a DOE (ASCR) and an NSF (Engineering Design) Early Career awards, as well as multiple teaching awards from CU Boulder and AIAA. His research interests include: Uncertainty quantification, data-driven modeling, optimization under uncertainty, and computational stochastic mechanics.
Realistic analysis and design optimization of complex engineering systems require not only a fine understanding and modeling of the underlying physics and their interactions but also recognition of intrinsic uncertainties and their influences on the quantities of interest. Uncertainty Quantification (UQ) attempts to address the latter issue; it aims at a meaningful characterization of uncertainties from the available measurements, as well as efficient propagation of these uncertainties through the governing equations for a quantitative validation of model predictions. The development of efficient uncertainty propagation strategies for complex engineering systems involving multi-scale and/or multi-physics phenomena is a subject of growing interest in UQ.
I will start this talk with a brief discussion on challenges associated with UQ of complex systems and a high-level introduction to recent work performed by the UQ Group at CU Boulder to tackle these challenges. I will then focus on model reduction approaches for efficient uncertainty propagation and optimization under uncertainty. At the core of these techniques is a systematic use of models with different levels of fidelity, e.g., coarse vs. fine discretization of the same problem, that lead to considerable improvements in performance of standard UQ techniques. During the talk, I will present application examples to highlight the efficiency of these multi-fidelity model reduction approaches and their wide applicability to a broad range of problems.
Amber Samaniego
a2samaniego@ucsd.edu