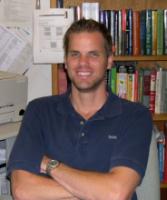
Michael Todd received his B.S.E. (1992), M.S. (1993), and Ph.D. (1996) from Duke University's Department of Mechanical Engineering and Materials Science, where he was an NSF Graduate Research Fellow. In 1996, he began as an A.S.E.E. post-doctoral fellow, then a staff research engineer (1998), and finally Section Head (2000) at the United States Naval Research Laboratory in the Fiber Optic Smart Structures Section. He joined the Structural Engineering Department at the University of California San Diego in 2003, where he currently serves as Professor. To date, he has published over 140 journal papers, five book chapters, over 350 conference papers and proceedings, and has 4 patents. His main research areas are in applying nonlinear time series techniques (such as chaotic interrogation) to SHM problems and stochastic modeling/uncertainty propagation for SHM applications, among many others.
Damage prognosis is the act of making an informed prediction of the future state of health of a system or component, given that a current diagnostic state is available with its uncertainty quantified. It has been noted that several material failure modes occur, after initiation phases, as a consequence of a positive-feedback mechanism. Positive feedback systems continually “accelerate” their underlying physics until failure, and as such, are unstable processes that result in the tendency of the rate of change in the observable data (or more generally, damage-sensitive features) to approach infinity. Models have been proposed based on this asymptotic property of positive feedback mechanisms for predicting the time to criticality, generally now known as the “failure forecast method”. This approach links the structural health monitoring process, which results in the features representative of the current state of performance, to a predictive process whereby the features are projected to a self-defined time of criticality, defined by the asymptotic property of the model. The typical implementation of this approach is to compute the inverse time rate-of-change in the features and linearly regress that data vs. time; inevitable uncertainties in the data, measurement process, or environmental contamination noise will corrupt the regression, leading to distributions in the parameters used to make the failure forecast. This study will look at a parametric implementation of the failure forecast method using a probability density function computed from the regression process and evaluating its predictive performance on fatigue data, considering regression window, sampling time, noise level, and predictor. Comparisons will be drawn in predicting a fatigue failure with conventional inspection strategies, revealing that continuous structural health monitoring provides generally much greater confidences in making failure forecasts.
Amber Samaniego
a2samaniego@ucsd.edu