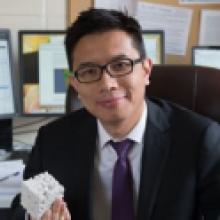
Dr. Sun is an associate professor in the department of civil engineering and engineering mechanics at Columbia University. He obtained his Ph.D. from Northwestern in 2011 and worked as a senior member of technical staff at Sandia National Laboratories from 2011 to 2013. His research focuses on theoretical, computational, and data-driven mechanics for multiphase materials with complex microstructures. He has published over 70 journal articles on computational mechanics and is the recipient of several awards including the IACM John Argyris Award, ICE Zienkiewicz Medal Prize, the ASCE EMI da Vinci Award, the NSF CAREER award, Young Investigator Awards from the Army Research Office and Air Force Office of Scientific Research.
We present an adaptive hybridized model-based/model-free strategy that ensures the feasibility of multi-physics simulations when different amounts of data are available. In the data-rich situation, we extend the data-driven approach where the hydraulic and solid constitutive laws may switch from model-free to mode-based depending on the availability of data. Techniques related to identifying the constitutive manifold, the influence of the normed space, and the distance-minimized algorithm accelerated by the K-dimensional tree search will be discussed. In the cases where experimental data are less abundant, we build interpretable macroscopic surrogate elasto-plasticity models inferred from sub-scale direction numerical simulations (DNS) or experiments with limited data. To simplify these training processes and improve the interpretability of the trained models, we break down the training of material models into multiple supervised machine learning programs for elasticity, initial yielding, and hardening laws that can be conducted sequentially. To handle pressure- and rate-dependence of the plastic responses, we reformulate the Hamilton–Jacobi equation such that the yield function is parametrized in a product space spanned by the principal stress, the accumulated plastic strain, and time. To test the versatility of the neural network meta-modeling framework, we conduct multiple numerical experiments where neural networks are trained and validated against (1) data generated from known benchmark models, (2) data obtained from physical experiments, and (3) data inferred from homogenizing sub-scale direct numerical simulations of microstructures.