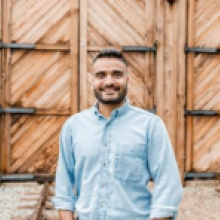
Dr. Mayank Chadha is currently an Assistant Adjunct Professor in Structural Engineering at UC San Diego. He teaches courses in mechanics and Structural Health Monitoring. Most of his academic efforts focus on research in applied mechanics, SHM, and application of machine learning to engineering problems. His research interests span various areas, such as large deformation beam theories, building forecasting models for battery life prediction and river discharge forecasts, Structural Health Monitoring (SHM), Bayesian Statistics (including Bayesian model updating), and Bayesian optimization for optimal system design, as well as engineering decision theory. Apart from his academic activities, Mayank is an avid reader. He also dedicates time to managing his business ventures and occasionally creates content for his YouTube channel, “Doctor Chadha”.
With growing urbanization, aging infrastructure in the developed world, and the rapid expansion of infrastructure portfolios in developing countries, there is a demand for a modern and novel asset management framework. This framework should be underpinned by the science of SHM, empowered by digital technology and artificial intelligence. It must be capable of addressing the inherent uncertainties in complex systems and utilize state-of-the-art decision-making capabilities to ensure the seamless operation and safety of asset portfolios. At a global level, the primary objective of such a framework is: (1) to diagnose the structural state of assets in the portfolio (diagnostics) and to probabilistically forecast the future evolution of damage (prognostics); (2) to transform acquired information about the assets into actionable decisions; (3) to update the overall digital twin/cyber model when data is acquired or if there is any change to the system due to actions. Building this asset management framework, which involves numerous complex decision-making processes, is a colossal task that necessitates multidisciplinary research. This research includes physics-based modeling, damage degradation analysis, Bayesian model updating, system optimization, uncertainty quantification, and decision-making, among other areas. The focus of this presentation revolves around this framework and its associated capabilities.