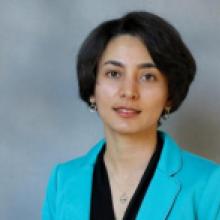
Maryam Shakiba is an assistant professor at the Civil and Environmental Engineering Department at Virginia Tech. Before joining Virginia Tech, she was a Postdoctoral Research Associate at the University of Illinois at Urbana-Champaign. She received her Ph.D. from Texas A&M University and her B.S. and M.S. degrees from Tehran Polytechnic. Shakiba’s group develops physics, chemistry, and mechanics-based constitutive equations to simulate multi-physics conditions for different advanced materials. The group also devises high-fidelity as well as mechanistic machine learning approaches to solve engineering problems. Our goals are to (1) develop theoretical frameworks to understand advanced material responses under extreme multi-factor conditions and (2) integrate the theoretical framework with machine learning approaches as physics-based machine learning is key to creating true digital twins. Shakiba is the recipient of the AFOSR young investigator program (YIP) award to investigate additively manufactured composites for high-temperature application and the NSF CAREER award (is recommended) to understand the mechanisms that cause fragmentation, foliation, and fibration in macrosplastics and generate microplastics.
This presentation discusses finite element and physics-based data-driven modeling of progressive damage in fiber-reinforced composites microstructure. We focus on two- and three-dimensional representations of additively manufactured composites. Such simulations are critical in order to design optimized composites for additive manufacturing. In fiber-reinforced composites, cracks initiate around the fibers aligned transversely to the loading direction. The transverse cracks can potentially cause leakage in specific applications or progress to inter-ply delamination and catastrophic failure. We, first, integrate an efficient numerical framework with robust and accurate constitutive equations to study transverse behavior and multiple cracking of two-dimensional representations of fiber-reinforced composite laminates. We then extend our simulations to three-dimensional representations of additively manufactured discontinuous fiber-reinforced composites. The representative volume element is generated randomly, based on a target distribution for the fibers' aspect ratios reported by experiments. The developed frameworks are validated versus experimental results. The effects of several material properties on the transverse crack density, delamination, intra-ply stress redistribution, and damage propagation are then investigated. Since finite element simulations of such composites are complex and computational cost is expensive, we develop a deep learning framework to predict the post-failure full-field stress distribution and the crack pattern in two-dimensional representations of the composites based on their microstructures. The deep learning framework contains two stacked fully-convolutional networks. A physics-informed loss function is also designed and incorporated to improve the proposed framework's performance further and facilitate the validation process. The accuracy of the deep learning framework to predict the complex nonlinear behavior is obtained to be more than 90%.