Structural Health Monitoring, Prognosis, and Validated Simulations Projects
Principal Investigator(s):
Michael Todd
Summary:
The field of additive manufacturing (AM) is a quickly- growing area of research and industrial development, experiencing double-digit annual growth percentage rates, and currently valued at an estimated $9 billion. AM enables the incorporation of complexities within parts such as internal cooling channels, lattice structures, and topology optimized features which can be impossible to fabricate with traditional, subtractive techniques. Most present quality control (QC) approaches for metallic additive manufacturing are performed post-production, including ultrasound, thermography, penetrant testing, eddy current, and x-ray computed tomography and provide either quality assessment after the part is already fully constructed, resulting in wasted fabrication time and materials if the part does not pass inspection. More importantly, the access of these inspection techniques is limited to the external faces of the manufactured part, making the quality of the internal features unknown, which may have been the driving reason for the part to be produced by AM.
We are developing a structured light sensing technique specifically tailored for in-situ part monitoring in additive manufacturing. This technique is used to measure layer- by-layer features such as powder coating layer health, sintered metal layer height, porosity, and overall part dimensions. The sensing method works by projecting patterns of light into the part chamber, and capturing images which are combined to create a high resolution height map. The sensing method will be used for closed feedback communication integrated into the print routine.
We are also developing a statistical model which estimates the uncertainty of each height measurement point from noise statistics on the input (pixel intensity). This model will ultimately be used in the closed feedback decision making routine, allowing for statistical confidence levels of build-terminating defects.
We are currently reaching out to a variety of companies in the AM industry to test the sensing technique. On the schedule is a test with an on-campus start-up, Additive Rocket Corporation, where we plan on performing initial height measurements on powder coating layers, and powder coating defects such as clumping and streaking.
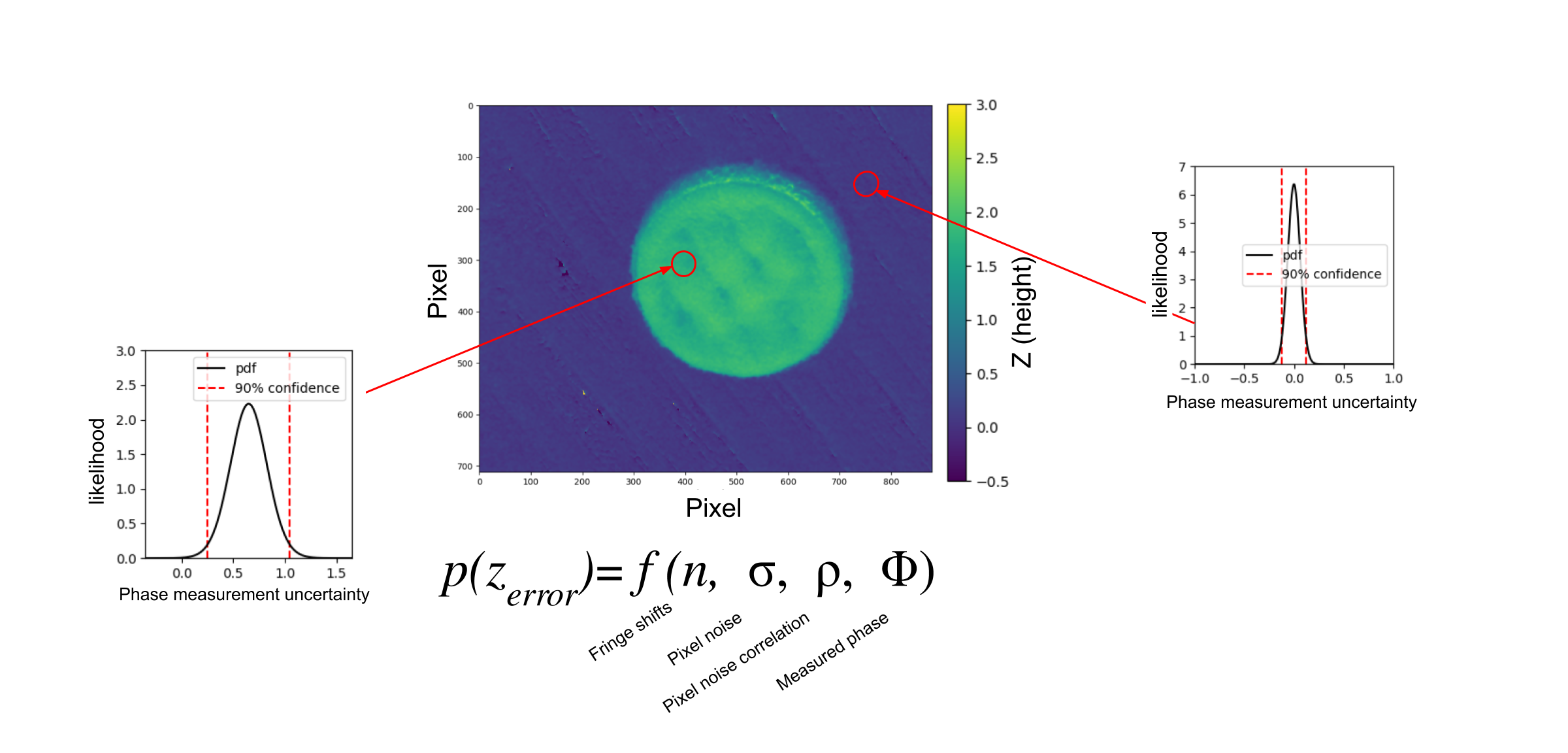
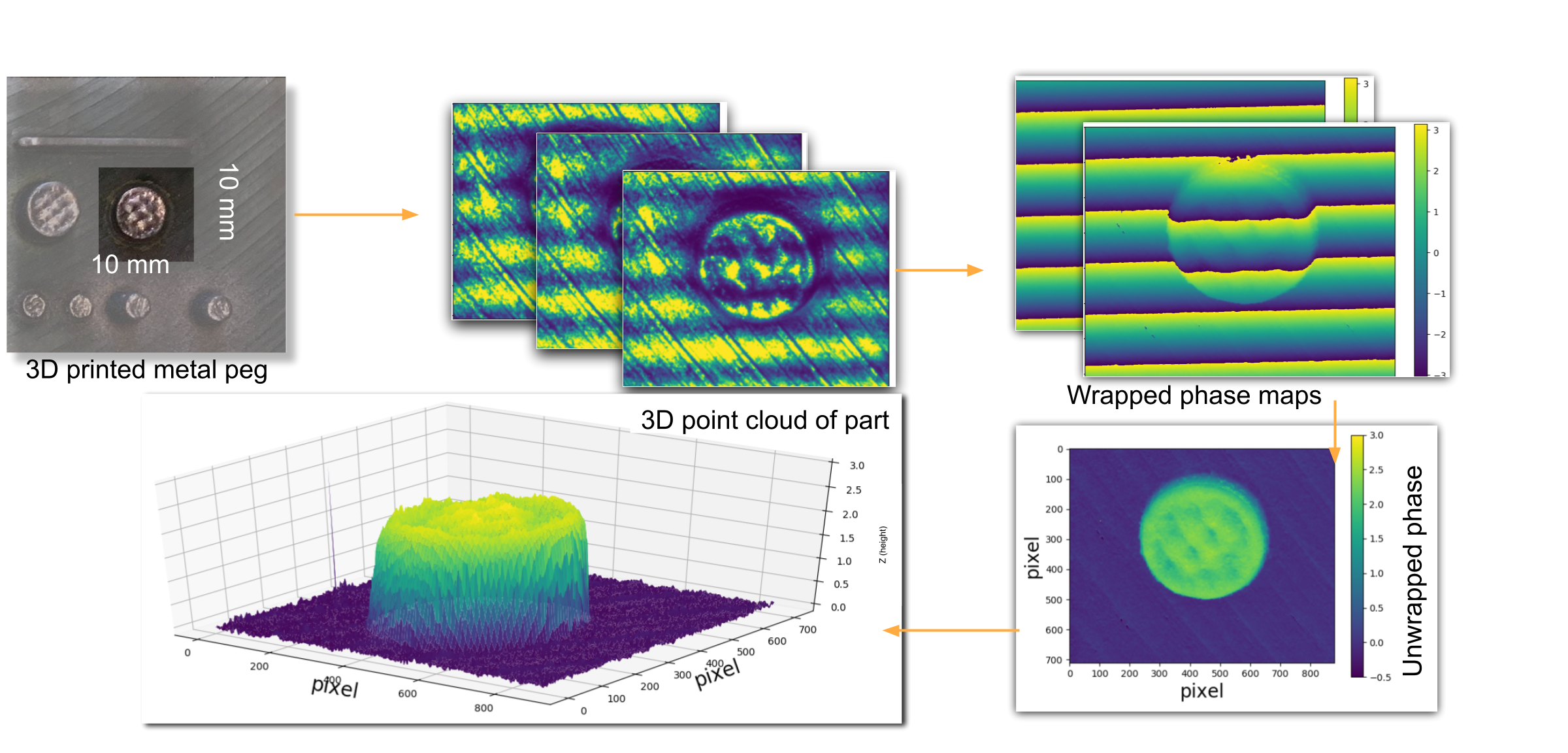
Principal Investigator(s):
Michael Todd
Summary:
Operational condition assessments are frequently used by field engineers to assess inland navigation assets and components. These assessments are reported using a discrete rating system. The goal of these assessments is to initiate effective risk- informed budget plans for maintenance and repair. However, some of the components in the navigation infrastructure (such as navigational locks) cannot be inspected when the water is at operational levels. In many of these cases, ratings due to protocols are sometimes given to components even when they are not inspected. This leads to highly abstracted inspection data. On the other hand, navigational locks are equipped with structural health monitoring (SHM) systems to achieve effective and high-precision real-time damage diagnosis through online monitoring data. UCSD has developed a novel damage prognosis and maintenance planning framework for miter gates, one of the US Army Corps of Engineers (USACE) most important structural assets, by integrating highly abstracted inspection data with physics-informed simulations.
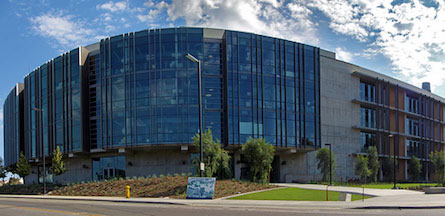
Principal Investigator(s):
Joel Conte
Researcher(s):
Mukesh Ramancha
Summary:
A promising approach for performing SHM/ DP of civil infrastructure systems is through utilizing a calibrated finite element (FE) model. This involves collecting measurement data using heterogeneous sensor arrays (which may consist of accelerometers, GPS- based displacement sensors, LVDTs, string potentiometers, strain gauges, etc.) deployed on the system. Then, a FE model of the system is developed from design or as-built drawings of the system and using explicitly formulated assumptions and hypotheses. Due to the inherently complex nature of civil engineering systems and due to the presence of wide-ranging uncertainties, it is extremely difficult to model these systems accurately. Often, FE models contain parameters that are unknown or known with significant uncertainty (e.g., inertial, damping, hysteretic material law, loading, boundary conditions, and geometric parameters). The process of FE model updating involves estimating the unknown parameters of the FE model accounting for numerous sources of real-world uncertainties (i.e., noisy output measurements, unknown/ partially known/noisy input measurements, uncertainty in FE model parameters, FE model form uncertainty) and probabilistically characterizing their estimation uncertainty. When a mechanics-based nonlinear FE model (able to capture the damage and failure mechanisms of concern) of the system is updated, it can be directly used to accurately or comprehensively perform damage detection, localization, classification, severity assessment and prognosis. Our lab is working on SHM/DP of concrete dams, miter gates, and bridge structures.
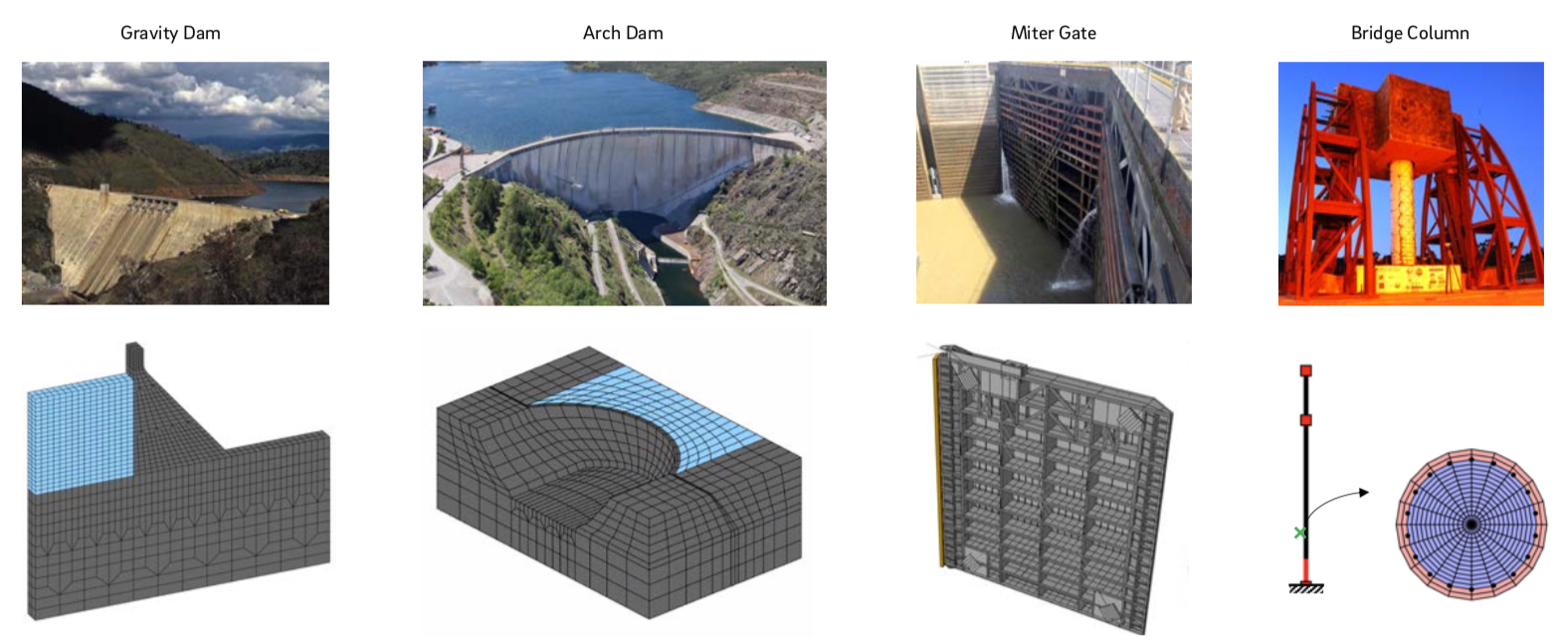
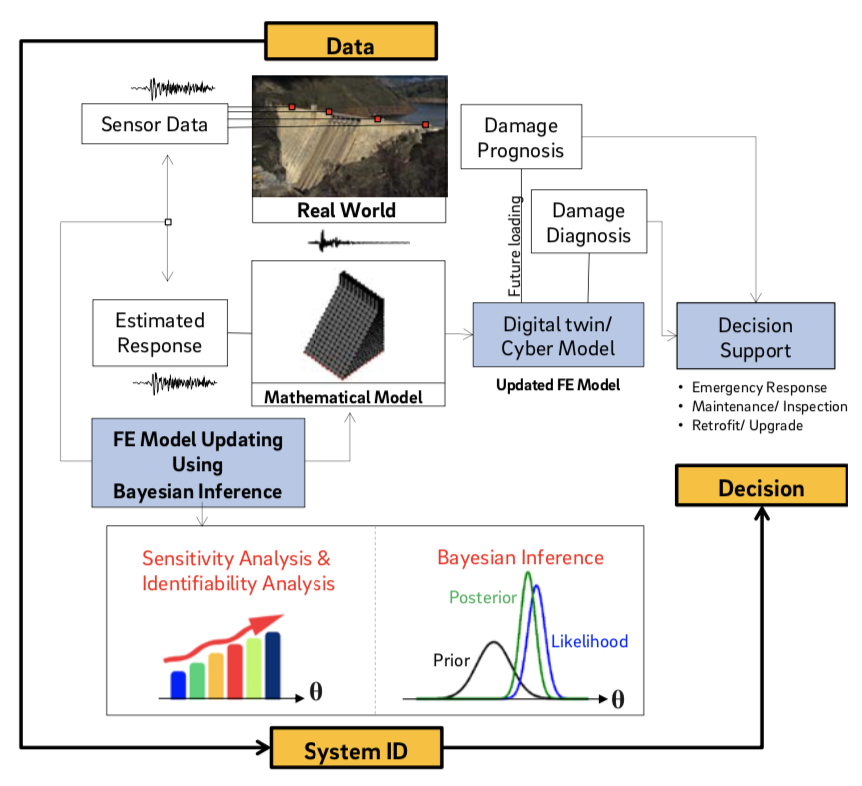
Principal Investigator(s):
Francesco Lanza Di Scalea
Summary:
Internal defects in rails cause a number of train accidents worldwide, including derailments. Current rail inspection systems use ultrasonic transducers hosted in fluid-filled wheels to detect internal cracks before they reach critical size. These systems are operated at a maximum speed of 25-30 mph by dedicated inspection vehicles that need to be scheduled during normal train operations.
Under Federal Railroad Administration (FRA) funding, UCSD is working on a radically new method to inspect rails that can enable “smart trains” to conduct the inspection at regular traffic speeds (80 mph and beyond). The approach is based on the idea of passive reconstruction of an acoustic transfer function between two points of the rail by cross-correlating (and opportunely normalizing) apparently-random measurements of dynamic excitations naturally occurring in the rail due to the rotating wheels of a traveling train. A system based on this idea was designed and constructed using pairs of non-contact air-coupled acoustic receivers. Special signal processing algorithms are being developed to increase the stability of the passively-reconstructed transfer function, i.e. minimize the variance and bias of the transfer function’s estimate. A prototype has been tested at the Transportation Technology Center (TTC) in Pueblo, CO, the premiere testing facility in the country for railroad engineering research. For these tests, the UCSD prototype was mounted underneath the FRA DOTX216 test car. Very promising results were obtained at speeds up to 80 mph, with positive identification of rail discontinuities (joints, welds , defects) from changes in the passively-reconstructed transfer function solely using the train wheels as the dynamic excitation of the rail.
Sample of inspection results at 80 mph test speed (TTCI RTT track)
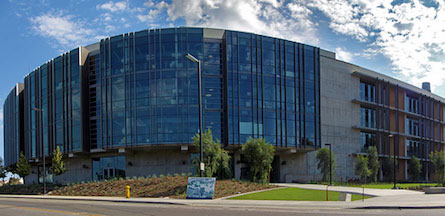
Principal Investigator(s):
Michael Todd
Summary:
The primary objective of the structural health monitoring (SHM) system is to continuously monitor the state of the structure and evaluate the structural integrity at any time. Minimizing the losses due to failure, and the maintenance cost in the real-world structures requires the installation of an efficient and optimized SHM system. The first part of this research focuses on developing a framework for optimal sensor network design using Bayesian optimization concerning SHM applications using Bayes risk as an objective function. Bayes risk considers the costs of consequences associated with making decisions and design selection (extrinsic cost) in the monitoring process, as well as intrinsic costs (e.g., sensor costs and their maintenance costs), and hence, is a natural choice for the objective function. The optimal sensor network is obtained by minimizing Bayes risk. Solving this problem involves using advanced techniques of Bayesian inference, surrogate modeling using Gauss process regression (GPR), uncertainty quantification, and Bayesian optimization
The second part of this research is to arrive at the state of the structure, like to decide if the structure is damaged or undamaged, depending on the decision-maker’s individual or organizational behavioral risk-profile using expected utility theory. Usually, SHM provides the distribution of the state-parameter from which the structural state is determined in probabilistic terms. The subjective actions corresponding to the state of the structure then consist of various maintenance strategies (like to inspect or not to inspect) that has an associated cost to it. This paper tackles the situation where the state of the structure is subjective to the decision maker’s risk profile and is dependent on the uncertain state parameter (like crack length, and thickness of corrosion). It attempts to answer the question: Given the risk profile of the decision maker and the distribution of the state-parameter, which structural state must be chosen so that the consequence or the losses are minimized in an average sense? This involves understanding and designing individual and organizational risk-profiles, using the concept of the value of information, prior, posterior, and preposterior decision analysis. Both these investigations are applied to a miter gate problem.
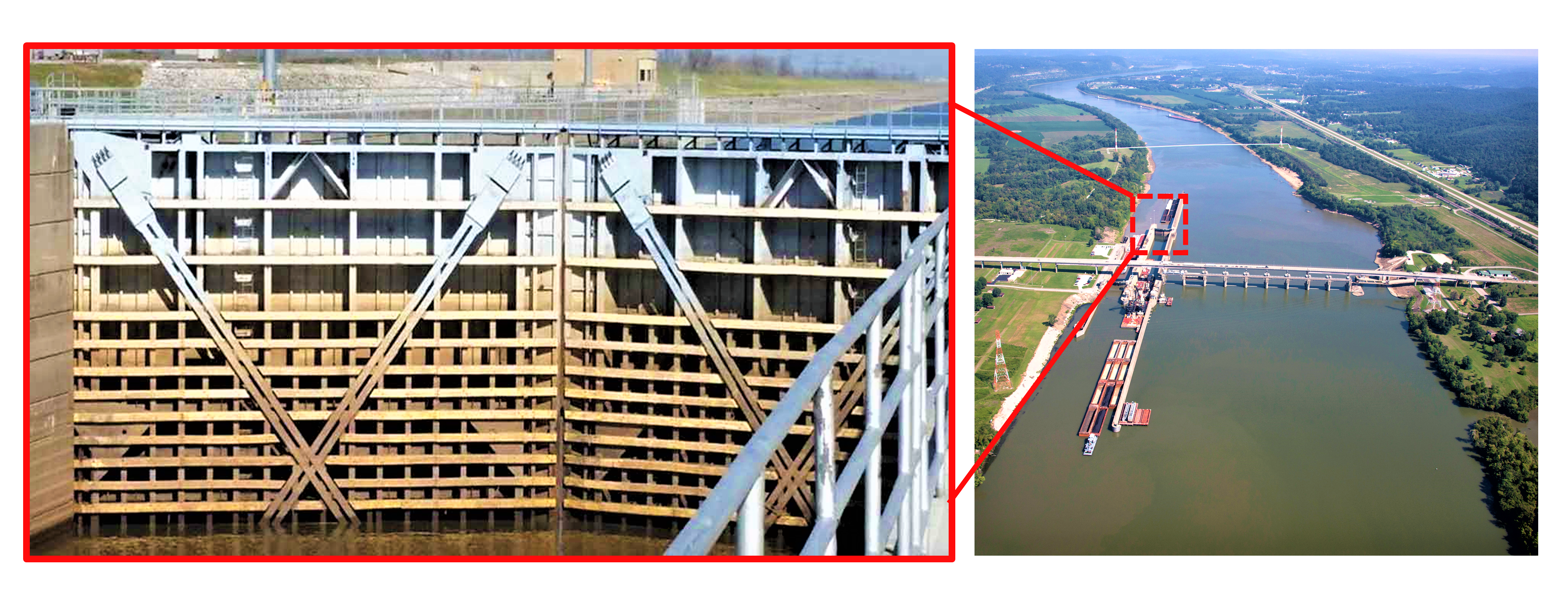